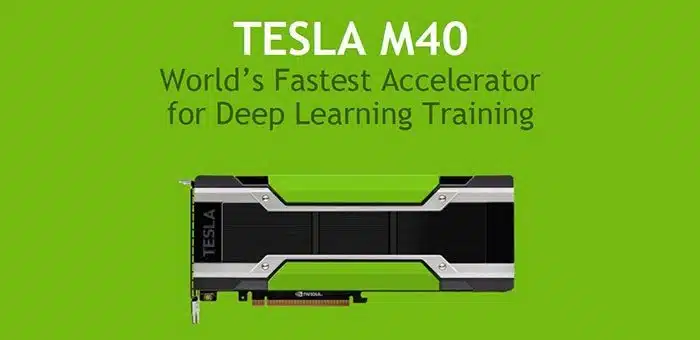
Machine learning is a process whereby a model is developed based on input data then trained to use that data to predict how new inputs will affect the model.
The model learns with each new scenario. The accuracy of its predictions is based on the parameters set. If the model’s parameters are tweaked to favour a particular prediction then it will flag another prediction that it previously considered correct as being wrong. This means that the person setting the program must run through numerous iterations to find the right balance to keep the predictions accurate over a wide scope of input data.
Deep Learning
Deep learning is a revolutionary branch of machine learning research that brings the field even closer to technology developers’ dreams of artificial intelligence. Deep learning algorithms are designed to mimic how our brains work in the neocortex where 80% of our thought processes occur. The software learns based on sounds, images and other input data and then changes its own algorithms to recognize what those inputs mean, much in the same way that our brains create new neural pathways for new experiences.
The premise for deep learning has been around for decades. What has held software developers back from creating intelligent computers is the limitations of computing power and mathematical knowledge. After a series of advancements and setbacks, computer scientists now have the know-how and the equipment to model virtual neurons in more layers than ever before.
Google is one company deeply invested in helping the advancements of deep learning. They shelled out nearly $600 million dollars to acquire DeepMind, a research group at the forefront of deep learning developments.
These developments in deep learning have paved the way to advancements in speech and facial recognition. Using these advancements, Google was able to develop an algorithm that was 100% better at recognizing images such as cats in YouTube videos and increase the efficiency of the speech recognition on Android phones.
The Technology That Makes it Possible
Deep learning development has been around since the 70s but a slow-down in processing power advancements in the 80s all but froze the program. The main proponent that has accelerated the development of deep learning algorithms in recent times is the developments of powerful compute devises such as graphics processor units such as NVIDIA’s Tesla Range. The power of GPUs has enabled developers to cut down on the amount of time that the machines take to train. They have cut training time down from weeks to hours.
GPUs made their name powering gaming computers. NVIDIA, the company that dominates the GPU market, is synonymous among gamers with quality graphics and stellar frame-rates however the potential of GPUs does not stop there. They are also used for facial recognition software on Facebook as well as the vehicle detection technology that keeps Audi’s driverless cars from running into each other.
The models that lead the pack in terms of raw power are 8x GPU rackmount servers such as our HPC-R2220-U2-G8. The people at NVIDIA designed it to be the worlds’ most powerful data centre accelerator, specifically to aid deep learning algorithms.
The Artificial Brain
There have been many comparisons between deep learning programs and brain function however researchers are quick to point out that the two are still very different. Deep learning software may learn a language the way a child would however, it will not go out to look for new information and experiences.
Applying deep learning to applications that not only recognize speech and recognition but also manipulate the data for its own output is limited by the power of the processors available and how inputs can be managed.
Solutions to these problems include feeding the computer with a set of rules about how the world works. This takes a lot of time and even then the system may be unable to deal with the large amounts of ambiguous data. Programmers worked around this by training their neural networks to identify a certain input and relate it to a particular neuron. The prevalence of certain inputs led to certain neurons being assigned certain weights, much in the same way that our brains strengthen neural connections that are used often. The complexity of how many neural connections needed to be made sorely limited the complexity of the patterns that they could recognize.
The largest neural network to date boasts over a billion neural connections.
What is Next for Deep Learning?
Google hopes that the leaps and bounds that they are making in the field of deep learning will help them make their self-driving cars safer. That may be some way in the future so for now they are concentrating on making image searches on YouTube more efficient.
Deep learning has taken us that much closer to the utopian dream of artificial intelligence. Unfortunately, modelling the human brain is a task with countless complexities therefore we may need to develop a few more techniques before we can perfect it.